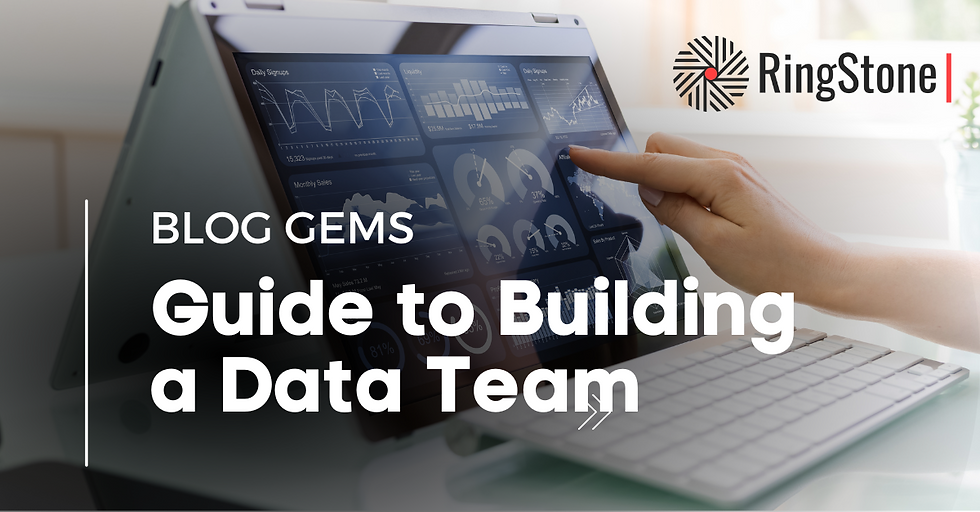
Introduction
The rapid evolution of technology and the increasing importance of data in decision-making have made building a strong data team more critical than ever. Whether you’re a startup looking to leverage data for growth or an established company seeking to optimize operations while staying compliant, having the right people in the right roles is essential. In this blog, we’ll break down the key positions and responsibilities vital to building a high-performing data team, offering guidance on structuring your team for maximum impact.
The Importance Of A Data Team
Data Teams Drive Business Growth By Transforming Complex
Raw Data Into Actionable Business Insights
Data has more impact than ever in today's business world, as it can add value to practically every aspect of an organization. Having a dedicated data team is crucial as they possess the expertise to gather, process, analyze, and interpret data, turning it into a valuable strategic asset. They drive business growth by transforming complex raw data into actionable business insights.
Maintaining a dedicated data team allows businesses to make informed decisions based on data rather than instinct. This enables leaders to develop strategies based on solid data and analysis, thereby increasing the likelihood of success. Furthermore, by accessing real-time insights from outputs such as reports, dashboards, or predictive modeling, businesses can understand customer behavior, operational issues, and evolving market conditions, leading to more agile and responsive decision-making.
Additionally, internal teams in organizations can benefit from the cultural impact that data teams bring. For instance, experimentation can enhance product development, leaders can better forecast and influence growth, and operational teams can find efficiencies through data insights.
Key Roles In A Data Team
Data Scientist / Data Analyst
Data scientists and data analysts play crucial roles in any data team. They are responsible for extracting meaningful insights from large volumes of data. They are typically experts in statistics, programming, and problem-solving. They use these skills to analyze complex datasets, identify patterns, and provide actionable recommendations.
These two job titles are frequently used by different businesses, yet they refer to similar responsibilities. For example, data scientists at Meta often lead product experimentation, whereas this typically falls under the remit of a data analyst at other companies. Generally, data scientists have more expertise in machine learning and predictive modeling, while data analysts typically specialize in reporting, building dashboards, product experimentation, and analyzing product releases.
Data Engineers
Data engineers are responsible for building and maintaining the infrastructure that allows data to be collected, stored, and processed efficiently. They often work closely with software engineers, platform teams, and product managers early on in the product development lifecycle to help plan how products should be built to ensure the right data is captured.
Data engineers have expertise in designing and developing pipelines that extract, transform, and load (ETL) data from various sources into data warehouses or data lakes, allowing data analysts and scientists to perform their analyses.
As organizations scale, it is essential to ensure that data is stored efficiently to control infrastructure costs while retaining necessary data.
Machine Learning Engineer
Machine learning engineers specialize in developing, training, and deploying machine learning models. They turn machine learning prototypes into scalable production systems, often enabling AI and ML product solutions to end customers.
They work on creating and optimizing machine learning algorithms that can process, and train on, large volumes of data, typically referred to as big data. They continuously monitor the performance of machine learning models, making adjustments as necessary to maintain accuracy and efficiency.
Head Of Data
This role can have varying names, such as VP of Data, Chief Data Officer, Data Lead, or Director of Data.
The Head of Data defines the organization’s data strategy. This involves setting long-term goals for how data will be leveraged to support business goals and objectives. They work closely with other senior leaders to ensure that the data strategy aligns with the overall business strategy, enabling data-driven decision-making at all levels of the organization.
The Head of Data oversees the recruitment, development, and management of the team. They have the final say over project resourcing and prioritization. They are also responsible for mentoring and developing team members, fostering a culture of continuous learning, and encouraging innovation within the data team.
Data Governance Officer / Chief Data Officer
The data governance officer ensures compliance with data management regulations, such as GDPR, by establishing policies and procedures for data handling and processing. They also identify potential risks related to data privacy and security and develop strategies to mitigate them.
Key Considerations When Hiring A Data Team
1. Technical Skills And Tool Proficiency
Identify the core technical skills required for each role. For instance, Data Engineers should have strong skills in SQL, ETL processes, and cloud platforms, while data analysts and scientists need proficiency in a programming language such as Python or R, machine learning frameworks, AB testing, and dashboarding tools. A take-home test is typically used to assess these skills.
2. Data Storytelling
Much of the value added by having a dedicated data team is the ability to translate unstructured, messy, and ad hoc data into a clear, insightful, and easily understandable set of actionable insights. Telling a compelling story from the data is key to driving business value. One way to test for this is to have candidates present the results of their technical test in a presentation tailored to non-technical stakeholders.
3. Communication Skills
Fostering a strong culture of data-driven decision-making requires buy-in from the organization. This is hard to do when insights are poorly presented or too complex for non-technical stakeholders to understand. Data teams that can speak at the company all hands, host workshops, and convey insights clearly, both verbally and in written formats, are most effective. Take note of candidates' communication skills, as these can be a great asset to the team.
4. Business Acumen
While assessing a candidate’s technical skills is essential, it’s equally important that they can tie their work back to business objectives. Strong business acumen is a valuable skill for employees who work closely with data, as it optimizes their impact on KPIs that define the team’s success. Ask candidates questions about your organization’s business model or industry to see if they can see the bigger picture.
5. Ethics And Security-First Mindset
It’s critical that data teams are mindful of data privacy and security when analyzing customer data. The right team will ensure that best practices are researched and implemented to build the proper foundation for data protection and compliance with data regulations. Note that this is particularly important to consider when hiring data engineers, as they have the most influence on how data, including customers’ personal data, is stored and processed.
6. Team Composition
It’s essential to have a diverse set of skills within the team to allow the execution of complex end-to-end projects. A mix of technical-heavy profiles (data scientists, engineers) and strategic and strong communicators (analysts) is more likely to lead to an overall high performing and agile team.
Where Should A Data Team Sit In An Organization?
Where the data team sits inside an organization often influences the team’s priorities. There are several common structures where a data team can sit, each with its advantages and considerations.
There Isn’t A “Right” Answer To Where The Team Should Sit In The Organization,
It Depends On Where The Team Can Add The Most Value.
Within The Product Team
This approach is most effective when the organization has a well-resourced team of product managers, designers, and UX researchers, as this provides more opportunities for leveraging data throughout the product development lifecycle. Additionally, this setup can help the organization achieve its goal of increasing product experimentation by AB testing products and features
Within A Business Team, Such As Finance Or Marketing
This situation is often seen in data teams that have a stronger focus on business intelligence skills. In this setup, the data team is frequently involved in activities such as growth and financial modeling, creating dashboards, analyzing marketing strategies, and setting company-wide objectives & objectives. As Head of Data, I have reported to several CFOs leading fundraising rounds, while my team has supported their specific groups of stakeholders.
Within Engineering
In some organizations, like LinkedIn, the data team is part of engineering and reports directly or indirectly to the CTO. Having observed this arrangement, I believe that Data and Engineering teams should collaborate as partners, each with separate reporting lines. This is because data teams thrive when they are integrated within core business teams, enabling them to be more closely aligned with strategy and operational execution..
Reporting to the CEO
Organizations with a high level of data maturity and company-wide data literacy often have the Head of Data on the Senior Leadership Team (SLT), contributing to company-wide strategy and execution.
I’ve personally worked in organizations with each of these setups. There isn’t a “right” answer to the question of where the team should sit in the organization, as it depends more on where the team can add the most value and the data maturity and literacy of the organization as a whole.
Aligning the Data Team with Business Goals
Understanding Business Needs
Data teams can often be overwhelmed by a large backlog of business questions to analyze. It’s important to prioritize initiatives based on the business's key drivers, such as customer satisfaction, revenue growth, or operational efficiency.
Cross-Functional Collaboration
It’s critical for data teams to work cross-functionally to ensure insights generated are informed, relevant, and actionable. Working closely with teams such as marketing, finance, sales, operations, and customer support ensures that the data team has the wider business context to inform their work. Very often, a data team’s “customers” are other internal departments in the business, so it’s important to build strong working relationships and understand their perspectives.
Set Clear Objectives & Key Results (OKRs)
As an internal service team, it can be hard to measure the success of a data team. One way to do so is to set data-driven OKRs that relate to data-driven decision-making and the adoption of data insights. For example, “All feature releases have a detailed measurement plan to determine success” or “Analysis of AB tests is shipped within a week of experiments ending.”
Note that it’s not a good idea to tie your team’s success to changes in business metrics that are out of their control. For example, a product team might release a feature, and the data team’s analysis clearly shows why it was a failure and provides actionable recommendations. The data team did their part here, despite the poor performing output metric.
Conclusion
Building a successful data team requires a mix of roles with specialized skills and responsibilities. Each role, from the strategic oversight of the Head of Data to the technical expertise of data engineers and machine learning engineers, plays a crucial part in harnessing the power of data. By understanding these essential roles and ensuring you have the right people in place, your organization can unlock the full potential of its data.
Where the data team sits within the organization can influence its effectiveness. Whether embedded within a product team, inside business functions like finance or marketing or reporting directly to the CEO, their position should reflect where they can add the most value. This strategic placement allows them to be more responsive to the needs of the business, ultimately amplifying their impact.
Finally, each business has its own unique goals and challenges. When data teams understand the organization's key objectives, they can prioritize their work to focus on the insights that matter most. This alignment ensures that data-driven decisions support the overall strategy and help the company stay competitive in an increasingly data-centric world.
About The Author
Chris Snipp is an accomplished data leader with 10 years of experience in data analytics, financial modeling, and fundraising spanning multiple industries across European and US markets. He holds a BSc in Mathematics from the University of Bristol and specializes in product data analytics, business intelligence, and strategy.
Chris has held several leadership positions throughout his career, where he has been instrumental in scaling startups from their founding stages to those scaling beyond $1 billion valuations.
Passionate about leading high-performing teams, Chris excels in hiring, training, and mentoring individuals to drive data-driven decision-making within organizations. He is also experienced in planning and executing company strategy, supporting CEOs and CFOs through critical business phases. Contact Chris at chris.snipp@ringstonetech.com.