Data-Driven Decision Making: How to Turn Analytics into Business Growth
- Chris Snipp
- Sep 5, 2024
- 7 min read
Updated: Mar 4
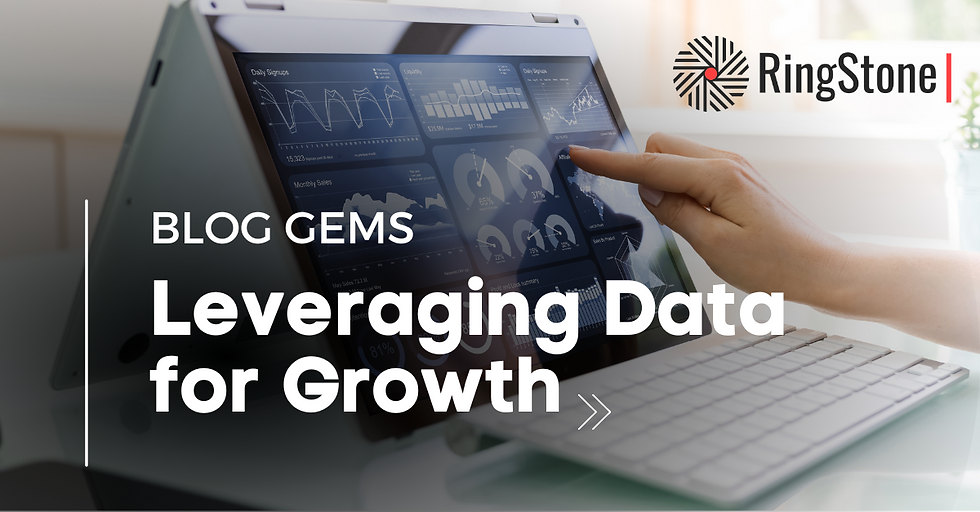
Introduction
In today’s data-driven world, the ability to transform raw data into strategic business decisions is more crucial than ever. Companies that harness the power of data analysis to uncover insights that can drive innovation, optimize products, and fuel business growth. In this blog, we’ll explore how to turn data into actionable strategies, guiding you through the essential steps of leveraging data analysis to make informed decisions that propel your business forward.
What Is Data Analytics, And Why Is It Important?
By Leveraging Data Analytics, Businesses Can Make Smarter, Data-Driven
Decisions For Product Development And Strategy
Put simply, data analytics helps identify what's working well in a product and organization, as well as areas that need improvement. It helps businesses make products that people want and use, which can boost product-market fit and bolster growth. By leveraging data analytics, businesses can make smarter, data-driven decisions for product development & strategy.
Steps To Leverage Data For Business Growth
1. Define Your Objectives
Start by asking what specific questions you need to answer and what goals your business aims to achieve. This clarity helps you focus on collecting data that directly supports your business needs and prioritize analysis in the most impactful areas. Key metrics to consider might include product usage, conversion rates, and customer satisfaction scores.
One of the first things I would do when joining an organization is to construct a "metric tree" to visually represent all the business's key performance indicators (KPIs) and show how they are interconnected. For instance, in subscription-based businesses, where retaining customers is crucial for growth and revenue, even slight changes in conversion rates or early customer retention can significantly impact overall growth. Without a comprehensive understanding of these metrics, you’re essentially flying blind.
2. Collect The Right Data
It is important to ensure that you are collecting accurate data on all points of the customer experience.
Partner With Product Managers And UX Designers: They are usually experts in all the contact points your customers have with your product. As a result, they are valuable partners in identifying all the opportunities to gather data.
Cross-Check Data From Multiple Sources To Ensure Accuracy: For instance, you can compare financial transactions with your product’s subscription data to ensure that any assumptions around customer lifetime value (LTV) or average order value (AOV) are correct.
Every team in an organization can contribute to this process. For example, the engineering team can implement event-tracking to ensure that data is recorded every time a user interacts with the product. In addition, the finance team can integrate their systems with tools, such as Xero, to allow product teams to establish connections and gather transactional data.
3. Turning Data Into Actionable Insights
Companies with a dedicated data team are more likely to extract the most value from their data. The process of transforming raw data into actionable business insights involves several steps. Technical expertise and experience can make this process more efficient and lead to more significant insights.
Analyze The Data To Identify Significant Trends: Look for correlations, recurring themes, or outliers that indicate underlying issues or opportunities. This step is about making sense of the data and understanding what it’s telling you.
Asking Questions Related To Your Objectives: For example, "Why are users dropping off at this stage of the funnel?" or "What features are driving the most engagement?" These questions guide your analysis and help focus on the most impactful areas.
Prioritize Insights That Have The Highest Potential Impact On Business Goals: Not all insights are equally valuable. This involves evaluating the feasibility, cost, and potential return of acting on different insights.
Translate Insights Into Specific Actions That Can Be Implemented: For example, if data shows that users are dropping off during checkout, an actionable step might be to simplify the checkout flow or add additional payment options. Each insight should lead to an explicit, concrete action.
4. Implementing Changes Based on Insights
Engage All Relevant Stakeholders: Gain buy-in by involving key stakeholders early in the process. Address their concerns and ensure they understand the benefits of the proposed changes.
A/B Testing Different Approaches: With a backlog of new product features and ideas, you can experiment with new products and features through testing to minimize risk and measure impact accurately.
5. Measuring The Impact
After implementation, continuously monitor the results to see if the changes have the desired effect. Real-time dashboards can be a great way to do this, as they prevent the need for analysis to be regularly redone. If the initial actions don’t yield the expected results, be prepared to iterate. Revisit the data, refine your approach, and try again. Don’t underestimate the value of key learnings, even if they are a result of false hypothesis.
Real-World Examples
Product Teams That Deeply Understand Usage Build Better Products
1. Increased Conversion Through Onboarding Optimization
Analyzing each step of your onboarding process helps identify bottlenecks in the user journey that may prevent more potential customers from converting into paying customers. Understanding the performance at each step allows you to prioritize which parts of the customer onboarding journey need optimization the most. Funnels often have room for improvement; even small changes can lead to long-term business growth.
2. Enhanced Product Features Based on User Behavior
By tracking usage patterns and behaviors, companies can determine which features are most effective in keeping customers engaged and extracting value from the product. This can lead to increased investment in the most popular features, as well as improvements in areas with the most bugs.
3. Discovery Analysis Adds Data-Driven Feature Ideas To Product Roadmaps
Understanding how users interact with your product is critical for optimizing customer satisfaction, long-term retention, and the bottom line. As mentioned, it’s worth mapping out all of the key touchpoints that your user has with your product throughout their lifetime to understand drop-off points, “aha” moments, and drivers of value.
This can inform discovery analysis, which helps drive roadmap planning and prioritization. This allows for a far more data-driven product development process and culture. Product teams that deeply understand usage build better products.
4. Lower Churn Through Predictive Analytics
Retaining existing customers is often more cost-effective than acquiring new ones. By identifying at-risk customers early, businesses can focus their resources on retention efforts, saving money, and increasing customer lifetime value.
Predictive analytics can identify customers who are most at risk of churning, which gives companies an opportunity to address churn before it happens. This is often in the form of personalized offers and personalized CRM strategies.
5. Personalization Based On Customer Segmentation
Companies can use product data to segment their customers based on purchasing behavior, preferences, and demographics. By analyzing this data, the company can tailor marketing campaigns, product offerings, and customer service strategies to each segment. This can lead to higher engagement and conversion rates.
When customers use a company’s product, patterns can be analyzed, and they can be grouped based on things like their behaviors, demographics, and preferences. This can help an organization understand distinct patterns of how their product is being used, which can drive marketing strategies and product roadmaps.
6. Customer Support Insights Improve Efficiency & Inform Product Roadmaps
Many customer support solutions prioritize data, offering dynamic tools for tracking customer tickets and managing large support teams. Key performance indicators (KPIs) can monitor performance in areas such as resolution time for support tickets, efficient handling of ticket volume by support agents, and customer satisfaction with resolutions.
A key use case of this data is that product teams can analyze which issues are reported most frequently by users. This allows dedicated sprints to be allocated to fix bugs and release new product features to address them.
7. A/B Testing Optimizes User Experience & Minimizes Risk
Optimizing user experience is essential for any successful product, and A/B testing is crucial in this process. By systematically testing different versions of a feature and comparing them against a control group, statistical methods can tell us, with a certain degree of confidence, how much a feature change has driven a change in a KPI. This approach enables teams to make informed decisions about which changes to implement for all users and which to roll back, reducing the risk of releasing a poorly performing product or feature to all users.
8. Growth Modeling Drives Data-Driven Product Prioritization
Growth modeling is a powerful tool that drives product prioritization by clearly understanding the key drivers of revenue or growth for a business. Specifically, how different features, updates, or strategies can impact key business metrics like user acquisition, retention, and revenue growth. By simulating various scenarios and projecting their outcomes, growth modeling allows product teams to prioritize initiatives most likely to drive meaningful growth. This approach ensures that resources are allocated to features and improvements that offer the highest potential return on investment.
Conclusion
The True Power Of Data Lies In Its Application: Turning Analysis Into Action
In an age of abundant data, the ability to distill it into meaningful insights and actionable strategies can add huge value to a business. The process starts with a clear understanding of what data to collect and how it aligns with business objectives. Analyzing this data to uncover trends and opportunities provides the foundation for informed decision-making to propel a business forward.
But insights alone are not enough. The true power of data lies in its application—turning analysis into action. By implementing changes based on these insights and continuously measuring their impact, companies can stay agile and responsive to both internal needs and external market shifts. This ongoing cycle of data-driven improvement is essential for achieving long-term success and maintaining a competitive edge in the market.
About The Author
Chris Snipp is an accomplished data leader with 10 years of experience in data analytics, financial modeling, and fundraising spanning multiple industries across European and US markets. He holds a BSc in Mathematics from the University of Bristol and specializes in product data analytics, business intelligence, and strategy.
Chris has held several leadership positions throughout his career, where he has been instrumental in scaling startups from their founding stages to those scaling beyond $1 billion valuations.
Passionate about leading high-performing teams, Chris excels in hiring, training, and mentoring individuals to drive data-driven decision-making within organizations. He is also experienced in planning and executing company strategy, supporting CEOs and CFOs through critical business phases. Contact Chris at chris.snipp@ringstonetech.com.