History of Data
Living organisms have been processing data since the dawn of time. Most data is processed to support the organism's survival needs with situational and day-to-day decision-making. While most of this data is ephemeral, some data was captured and added to the collective intelligence. The latter was added to our DNA while other data was preserved through other means like cultural art and writings in more intelligent life forms.
The old model of data storage and processing, while adequate, has been generally a slow process and limited by the capabilities of the natural systems in place.
Most of the data we are accustomed to has been designed, organized, and optimized for human consumption until the dawn of technology, when things began to shift. Technology capabilities enabled the generation, storage, and processing of vast digital data. These are not easily consumable by humans, rather optimized for machines. The revolution also posed a challenge in mapping and translating the old data into the new model so it could be utilized.
Twenty years ago, data may have provided an edge to a business over another, but today it is a lifeline that impacts competitiveness, product quality, collaboration, ability to make decisions, go-to-market timeliness, and even cost management. Without a data processing arm, companies limit their access to critical business insights they can use to compete and make better decisions.
Modern successful businesses can accomplish three things with their data allowing them to leverage insights.
Quickly transition accumulated data from the old paradigm to the modern one.
Develop systems to continuously capture new relevant private and public data.
Use the data cycle effectively to translate data back to humans to make decisions.
7 Stages of Data
While most categorize data into 5 or 6 categories, we decided that seven stages are more appropriate, namely adding data Architecture and Destruction.

Data Architecture
Conceptual and architectural system design of the data types needed in the present and projecting future needs. This includes internal and external data and projecting both capacity and growth needs. It also includes the requirements or the key recurring questions and insights that a business needs on an ongoing basis.
“Data is like garbage. You’d better know what you will do with it before you collect it.” — Mark Twain.
Data Collection
This is about identifying the sources of data (e.g., internal systems, external systems, data lakes, data warehouses). This step includes data quality design and availability fault tolerance, ensuring high-quality data input and fail-over mechanisms. Ultimately, it is about collecting human and machine-readable data and storing them for processing.
Data Preparation and Cleansing
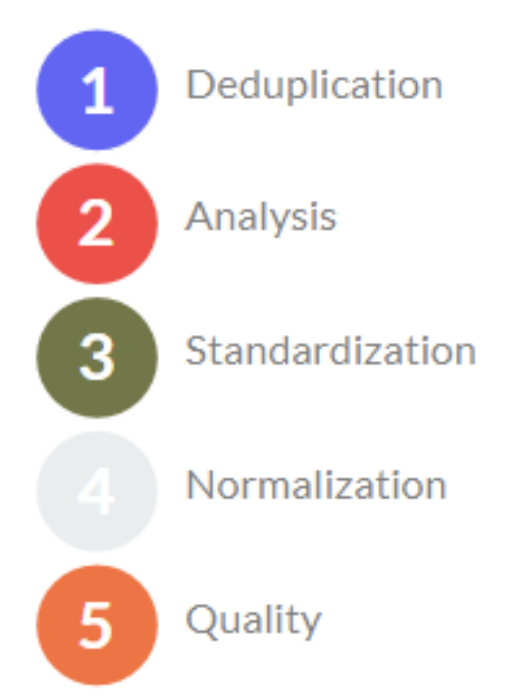
Data preparation is a lengthy cleansing and organization stage where raw data is diligently checked for errors, bad data, redundancy, completeness, and patterns. While often data scientists are performing this effort, a mature organization may already leverage algorithms effectively to do the bulk of the load, which reduces human error, time to completion, and surface patterns that humans are not able to easily deduce. This by the way, is statistically the least enjoyable part of the data science discipline. It’s tedious!
Data Aggregation and Propagation

Clean data is entered manually or preferably in an automated fashion and propagated to various destinations (e.g., CRM, SQL, RedShift) based on the architecture defined. Often there are limitations based on the tool choices and integration capabilities available. Data scientists and engineers devise automated software solutions to reduce effort and increase quality.
Data Exploration and Processing
This is the initial interpretation stage which is often a combined human and machine effort to explore relationships and pattern matching. Ideally, the processing is done using machine learning algorithms for efficiency.
Data Output, Interpretation, and Display
Clean digital computer-readable data is translated into simple human consumable insights. The end form can be graphs, videos, images, text, etc.
Data Storage and Destruction
Often some data that was not useful in the past may provide additional insights in the future that was not clear immediately, and new relationships can be explored. Data is intellectual property!
The storage, protection, and disaster recovery of data is critical for continuity. In addition, various compliance and ethical needs require data to be stored and protected.
The volume of data inevitably grows, and it is not feasible to save indefinitely. The decision to part with data is a difficult one, but sometimes necessary for compliance, cost, or end-of-life reasons. The challenge of data purging is to ensure that the data has been properly destroyed. Disposing of data is a careful process ensuring the integrity of the remaining data is intact.
The Data Advantage
Understanding the full data cycle and leveraging technology and professional data scientists in each team are critical to success in today’s business. The process of translating human and machine data to machine data and then back to human consumable information is science.
The various stages of data usefulness and importance to business decision-making in the illustration.
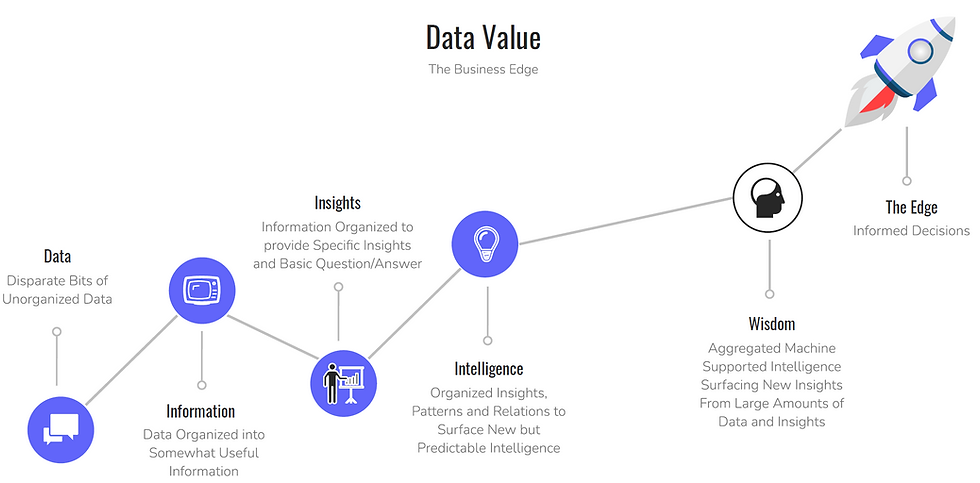
How do we use data at RingStone?
Data is our lifeline, and the entire business is architected and built around data. We use data to understand and continuously learn about the industry and measure quality and alignment during execution.
But most importantly, we recognized the need for our clients and how data can help companies and investors get ahead and get an edge.
RingStone Software collects anonymous benchmarks about the industry, best practices, and execution outcomes to help investors benchmark companies and investments. This provides consistency, quality, and measurable KPIs for our clients to be able to compare apples to apples when they need them. Our data insights and artificial intelligence get better with time and provides us with a unique competitive advantage. In addition, our software can also be licensed by investors or companies, providing measurable insights allowing them to track value creation plans progress and understand with precision where the technology stands inter portfolio company.
#duediligence #techduediligence #technicalduediligence #technologyduediligence #diligencechecklist #softwarediligence #ringstoneduediligence #vddduediligence #vdd #valuecreation
About the Author
Hazem has been in the software and M&A industry for over 26 years. As a managing partner at RingStone, he works with private equity firms globally in an advisory capacity. Before RingStone, Hazem built and managed a global consultancy, coached high-profile executives, and conducted technical due diligence in hundreds of deals and transformation strategies. He spent 18 years at Microsoft in software development, incubations, M&A, and cross-company transformation initiatives. Before Microsoft, Hazem built several businesses with successful exits, namely in e-commerce, software, hospitality, and manufacturing. A multidisciplinary background in computer engineering, biological sciences, and business with a career spanning a global stage in the US, UK, and broadly across Europe, Russia, and Africa. He is a sought-after public speaker and mentor in software, M&A, innovation, and transformations. Contact Hazem at hazem@ringstonetech.com