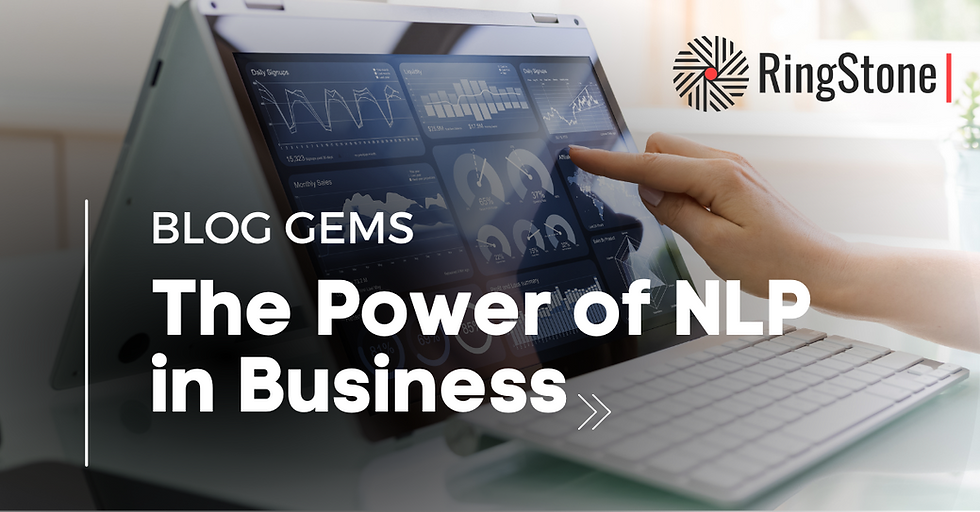
Introduction
In today's data-driven world, understanding, analyzing, and deriving insights from human language is more valuable than ever. Natural Language Processing (NLP), a subfield of artificial intelligence (AI), is at the forefront of this revolution, enabling machines to comprehend and interact with human language meaningfully. From virtual assistants to sentiment analysis and language translation, NLP has transformed how we communicate, work, and interact with technology. This blog explores the intricacies of Natural Language Processing, its definition, applications, industry insights, transformative impact on businesses and society, and how PEs and CTOs should think about NLP.
In This Article
What is Natural Language Processing (NLP)?
NLP Is A Branch Of Artificial Intelligence Focusing On The Interaction
Between Computers And Human Language
Natural Language Processing (NLP) is a branch of artificial intelligence focusing on the interaction between computers and human language. NLP encompasses a range of techniques and algorithms designed to enable machines to understand, interpret, and generate human language in a meaningful and contextually relevant manner.
At its core, NLP seeks to bridge the gap between human communication and computational analysis, allowing machines to process unstructured text data, extract valuable insights, and perform tasks that traditionally require human intelligence.
Applications And Use Cases
NLP-Powered Chatbots And Virtual Assistants Enable Businesses To
Provide Instant, Personalized Assistance To Customers
The applications of Natural Language Processing span various industries and use cases, demonstrating its versatility and transformative potential. In customer service and support, NLP-powered chatbots and virtual assistants enable businesses to provide instant, personalized assistance to customers, improving response times and overall satisfaction.
Sentiment analysis, another common NLP application, allows businesses to gauge public opinion, monitor brand perception, and identify emerging trends or issues in real time. Moreover, in healthcare, NLP facilitates the extraction of valuable information from medical records, clinical notes, and research papers, empowering healthcare professionals to make informed decisions and improve patient outcomes.
In finance, companies like JP Morgan Chase leverage NLP for fraud detection, risk assessment, and automated trading, enabling faster decision-making and more accurate predictions.
In the retail industry, e-commerce giants like Amazon use NLP to personalize product recommendations, optimize search functionality, and analyze customer reviews to improve product quality and relevance. Additionally, in the legal domain, NLP assists lawyers and legal professionals in contract analysis, case law research, and due diligence, reducing manual workload and increasing efficiency.
NLP Techniques
Natural Language Processing (NLP) encompasses a variety of techniques and applications designed to enable computers to understand, interpret, and generate human language. Here are some different types of NLP, along with examples to illustrate each.
Text Classification
Text classification involves categorizing or labeling text documents into predefined categories or classes. Examples include sentiment analysis (classifying text as positive, negative, or neutral) and topic classification (categorizing news articles into topics such as sports, politics, or entertainment).
Example: Classifying customer reviews as positive or negative to gauge sentiment towards a product or service.
Named Entity Recognition (NER)
Named Entity Recognition is identifying and classifying named entities (such as names of people, organizations, locations, and dates) in text documents. NER is commonly used in information extraction tasks, such as extracting entities from news articles or social media posts.
Example: Identifying and extracting the names of people, organizations, and locations mentioned in a news article.
Part-of-Speech Tagging (POS Tagging)
Part-of-speech tagging involves assigning grammatical categories (noun, verb, adjective, or adverb) to each word in a sentence. POS tagging is used in syntactic analysis, text analysis, and information retrieval tasks.
Example: Tagging each word in a sentence with its corresponding part of speech, such as "Noun (N)", "Verb (V)", or "Adjective (ADJ)".
Dependency Parsing
Dependency Parsing involves analyzing the grammatical structure of a sentence to determine the relationships between words. Dependency parsers identify syntactic dependencies between words, such as subject-verb relationships, object relationships, and modifier relationships.
Example: Analyzing the sentence "The cat chased the mouse" to identify the dependency relationships between words, such as "chased" being the main verb and "cat" being the subject.
Semantic Analysis
Semantic Analysis involves extracting the meaning or semantics of text documents to understand the intended message or context. Semantic analysis techniques include word sense disambiguation, semantic similarity measurement, and semantic role labeling.
Example: Determining the semantic similarity between two sentences, such as "The cat is chasing the mouse" and "The dog is chasing the cat".
Text Generation
Text Generation involves generating human-like text based on input data or prompts. Text generation models, such as language models and chatbots, use NLP techniques to produce coherent and contextually relevant text.
Example: Generating responses for a chatbot based on user input, using natural language understanding and generation techniques.
These are just a few examples of the different types of Natural Language Processing techniques and applications, each serving different purposes in analyzing and understanding human language.
Companies Leveraging NLP
Several leading technology companies and startups are at the forefront of leveraging Natural Language Processing to drive innovation and solve complex business challenges. Google's language processing capabilities power features like Google Search, Gmail's Smart Reply, and Google Translate, making information more accessible and actionable for users worldwide.
Amazon's Alexa and Apple's Siri utilize NLP algorithms to understand user commands, answer questions, and perform tasks, enhancing the utility and convenience of virtual assistants. Additionally, startups like OpenAI, founded by Elon Musk and others, are pushing the boundaries of NLP with advanced language models like GPT (Generative Pre-trained Transformer), enabling a wide range of applications, from content generation to language translation.
Google is a leader in leveraging Natural Language Processing across various products and services. Their language processing capabilities power features such as Google Search, enabling users to find relevant information quickly and accurately by understanding natural language queries. Additionally, Gmail's Smart Reply feature utilizes NLP algorithms to suggest contextually relevant email responses, streamlining communication and saving users time. Google Translate, another NLP-powered product, facilitates language translation, breaking down language barriers and making information more accessible and actionable for users worldwide.
Amazon
Amazon's virtual assistant, Alexa, relies on Natural Language Processing algorithms to understand user commands, answer questions, and perform tasks such as setting reminders, playing music, and controlling smart home devices. By integrating NLP into Alexa, Amazon has made voice-controlled technology more intuitive and convenient for users, enhancing the utility and accessibility of virtual assistants in daily life.
Apple
Apple's virtual assistant, Siri, leverages Natural Language Processing to understand and respond to user queries and commands. Siri can perform various tasks, including sending messages, making calls, setting reminders, and providing weather updates, all through natural language interactions. By integrating NLP into Siri, Apple has enhanced the user experience on iOS devices, making voice-controlled assistants a mainstream mobile technology feature.
OpenAI
OpenAI, a research organization co-founded by Elon Musk and others, is at the forefront of pushing the boundaries of Natural Language Processing. Their advanced language models, such as GPT (Generative Pre-trained Transformer), enable various applications, from content generation to language translation. By leveraging NLP technology, OpenAI empowers businesses and developers to automate tasks, generate creative content, and innovate communication capabilities.
Business Impact and Benefits
NLP Enables Businesses To Extract Valuable Insights From Vast Amounts Of Unstructured
Text Data, E.g., Social Media Posts & Customer Feedback
Adopting Natural Language Processing offers numerous benefits and business opportunities for organizations across various industries. By automating repetitive tasks, such as data entry, document processing, and customer inquiries, NLP enhances operational efficiency, reduces costs, and frees human resources to focus on more strategic initiatives.
NLP enables businesses to extract valuable insights from vast amounts of unstructured text data, such as social media posts, customer feedback, and market research reports, facilitating data-driven decision-making and targeted marketing campaigns. Additionally, by improving communication and interaction with customers, employees, and stakeholders, NLP strengthens relationships, fosters collaboration, and drives customer satisfaction and loyalty.
Here’s a summary of the top 10 benefits of utilizing NLP:
Improved Customer Service: NLP-powered chatbots and virtual assistants can provide instant, personalized assistance to customers, enhancing response times and satisfaction.
Enhanced Customer Insights: NLP enables businesses to extract valuable insights from unstructured text data, such as customer feedback, social media conversations, and reviews, facilitating data-driven decision-making and targeted marketing efforts.
Streamlined Operations: Automating repetitive tasks through NLP can streamline operations, reduce manual workload, and increase efficiency, leading to cost savings and improved productivity.
Personalized Recommendations: NLP algorithms can analyze user preferences and behavior to provide personalized product recommendations, improving customer engagement and driving sales.
Efficient Information Retrieval: NLP-powered search engines and retrieval systems can understand natural language queries, enabling users to find relevant information quickly and accurately.
Real-Time Sentiment Analysis: NLP enables businesses to monitor public sentiment, brand perception, and emerging trends in real time, allowing for proactive response and reputation management.
Risk Management: NLP can analyze vast amounts of textual data to identify potential risks, threats, and compliance issues, enabling businesses to mitigate risks and ensure regulatory compliance.
Enhanced Decision-Making: By extracting insights from textual data, NLP empowers decision-makers with valuable information and actionable intelligence, enabling more informed and strategic decision-making.
Language Translation and Localization: NLP facilitates language translation and localization, enabling businesses to reach global audiences, enter new markets, and communicate effectively across language barriers.
Competitive Advantage: Businesses that leverage NLP effectively gain a competitive edge by delivering superior customer experiences, optimizing operations, and capitalizing on emerging opportunities in the market.
How Should Private Equity (PE) Approach NLP?
PE Investors Should Approach Natural Language Processing As A Transformative Technology
With The Potential To Drive Value Creation And Competitive Advantage
Private equity (PE) investors should approach Natural Language Processing (NLP) as a transformative technology with the potential to drive value creation and competitive advantage across various industries. When evaluating investments in companies that utilize NLP, PE investors should consider several key factors to assess the technology's strategic fit, growth potential, and risk profile:
Market Opportunity: Evaluate the size and growth trajectory of the market segments where NLP is applied. Identify industries and use cases with significant demand for NLP-driven solutions, such as customer service, healthcare, finance, and e-commerce.
Differentiation and Competitive Landscape: Assess the company's competitive positioning within the NLP landscape. Look for proprietary technology, unique algorithms, or specialized domain expertise that differentiate the company from competitors and create barriers to entry.
Scalability and Growth Potential: Evaluate the company's NLP technology's scalability and ability to address evolving market needs. Consider product scalability, customer scalability, and geographic expansion opportunities to assess long-term growth potential.
Customer Value Proposition: Understand the value proposition of the company's NLP solutions from the customer's perspective. Assess how effectively the technology addresses customer pain points, improves operational efficiency, and drives tangible business outcomes.
Revenue Model and Monetization Strategy: Analyze the company's NLP offerings' revenue model and monetization strategy. Consider factors such as pricing strategy, subscription models, licensing agreements, and potential upsell or cross-sell opportunities to evaluate revenue growth potential.
Technology and IP Portfolio: Assess the strength of the company's technology and intellectual property (IP) portfolio related to NLP. Look for patents, trademarks, or proprietary algorithms that provide a competitive advantage and protect the company's innovations.
Team and Talent: Evaluate the company's leadership team, technical expertise, and talent pool in NLP-related disciplines. Consider factors such as domain knowledge, research capabilities, and track record of innovation to assess the company's ability to execute its NLP strategy.
Regulatory and Compliance Considerations: Consider regulatory and compliance considerations relevant to the company's NLP applications, especially in highly regulated industries such as healthcare and finance. Assess the company's approach to data privacy, security, and compliance with relevant regulations.
Partnerships and Ecosystem: Evaluate the company's partnerships and ecosystem collaborations within the NLP ecosystem. Look for strategic alliances with technology partners, academic institutions, or industry consortia that enhance the company's capabilities and market reach.
Exit Potential: Assess the potential exit opportunities for investments in companies that utilize NLP. Consider factors such as strategic acquisition interest from larger technology companies, potential IPO readiness, and market dynamics within the broader NLP ecosystem.
By considering these factors, PE investors can make informed investment decisions in companies that leverage NLP technology, effectively evaluating opportunities for value creation and sustainable growth in the rapidly evolving landscape of artificial intelligence and language processing.
How Should Chief Technology Officers (CTOs) Approach NLP?
Chief Technology Officers (CTOs) are critical in driving the adoption and implementation of Natural Language Processing (NLP) within their organizations. Here's how CTOs should approach NLP:
Understanding Business Needs: CTOs should start by understanding the specific business needs and objectives NLP can address. Whether improving customer service, automating repetitive tasks, or extracting insights from unstructured data, aligning NLP initiatives with business goals is essential for success.
Assessing Technical Feasibility: Evaluate the feasibility of implementing NLP within the organization's infrastructure and technology stack. Consider data availability, system integration requirements, and compatibility with existing software and platforms.
Identifying Use Cases: Identify potential use cases for NLP to deliver tangible value to the organization. This could include chatbots for customer support, sentiment analysis for market research, language translation for global communication, or text analytics for data mining.
Choosing the Right Tools and Technologies: Select the appropriate NLP tools and technologies based on the organization's requirements, budget, and technical expertise. Consider factors such as NLP libraries, frameworks, APIs, and pre-trained models that align with the organization's goals and capabilities.
Building or Buying: Decide whether to build NLP capabilities in-house or leverage third-party solutions and services. Evaluate the trade-offs between development time, resource requirements, and cost-effectiveness to determine the best approach for the organization.
Data Preparation and Quality: Pay attention to data preparation and quality assurance processes, as the performance of NLP models depends on the quality and quantity of training data. Ensure data is clean, labeled, and representative of the target domain to achieve accurate results.
Testing and Validation: Thoroughly test and validate NLP models and applications before deployment to ensure accuracy, reliability, and performance. Conduct rigorous testing across different datasets, languages, and use cases to identify and address potential issues or limitations.
Privacy and Security Considerations: Consider privacy and security considerations when working with sensitive or confidential data. Implement robust data encryption, access controls, and compliance measures to protect user privacy and mitigate security risks.
Monitoring and Optimization: Continuously monitor and optimize NLP models and applications to maintain performance and adapt to changing requirements. Implement feedback loops, performance metrics, and automated monitoring systems to identify and address real-time issues.
Educating Stakeholders: Educate key stakeholders, including executives, employees, and customers, about the capabilities and benefits of NLP. Foster a culture of innovation and collaboration, encouraging cross-functional teams to explore new opportunities and drive the adoption of NLP across the organization.
By approaching NLP strategically and systematically, CTOs can harness the power of language processing technology to drive innovation, improve efficiency, and unlock new opportunities for their organizations.
Conclusion
By Embracing NLP-Driven Solutions & Strategies, Organizations Can Gain A Competitive Edge,
Drive Operational Efficiency, & Deliver Great Customer Experiences
Natural Language Processing is a transformative technology key to unlocking new communication, analysis, and automation possibilities. From virtual assistants to sentiment analysis and language translation, NLP has permeated various aspects of our daily lives, revolutionizing how we interact with technology and each other.
As businesses and industries continue to harness the power of NLP, the potential for innovation and growth is limitless. By embracing NLP-driven solutions and strategies, organizations can gain a competitive edge, drive operational efficiency, and deliver great customer experiences in an increasingly digital and data-driven world.
Both Private Equity (PE) investors and Chief Technology Officers (CTOs) should approach Natural Language Processing (NLP) with a strategic mindset, recognizing its potential to drive value creation and innovation within organizations. For PE investors, evaluating investments in NLP companies requires a thorough assessment of market opportunity, differentiation, scalability, revenue potential, and regulatory considerations. By identifying companies with strong competitive positioning, differentiated technology, and robust growth prospects, PE investors can capitalize on the transformative potential of NLP to generate attractive returns.
CTOs implementing NLP within their organizations should focus on aligning NLP initiatives with business needs, assessing technical feasibility, identifying use cases, and selecting the right tools and technologies. By prioritizing data quality, testing, security, and ongoing optimization, CTOs can ensure the successful implementation and adoption of NLP solutions. Additionally, fostering a culture of innovation, collaboration, and stakeholder education is essential for maximizing the benefits of NLP and driving organizational growth and competitiveness.
In summary, PE investors and CTOs should view NLP as a strategic enabler of innovation, efficiency, and competitive advantage. By embracing NLP technology and leveraging its capabilities effectively, organizations can unlock new opportunities, enhance customer experiences, and drive sustainable growth in an increasingly digital and data-driven world.
As we embark on this journey of discovery and innovation, one thing is certain: Natural Language Processing will continue to shape the future of technology and redefine how we communicate, work, and live.
About The Author
Jon White is an experienced technology leader with over 34 years of international experience in the software industry, having worked in the UK, Malaysia, Bulgaria, and Estonia. He holds a BSc (Hons) in Systems Design. He led the Skype for Windows development teams for many years (with 280 million monthly connected users), playing a key role in the team's transition to Agile.
Jon has held multiple leadership positions throughout his career across various sectors, including loyalty management, internet telecoms (Skype), IT service management, real estate, and banking/financial services.
Jon is recognized for his expertise in Agile software development, particularly helping organizations transform to Agile ways of working (esp. Scrum), and is a specialist in technical due diligence. He is also an experienced mentor, coach, and onboarding specialist.
Over the last few years, he has completed over a hundred due diligence and assessment projects for clients, including private equity, portfolio companies, and technology companies, spanning multiple sectors. Contact Jon at jon.white@ringstonetech.com.