Large Language Models (LLMs): The AI Revolution in Action
- Victoria Steed
- Feb 13, 2024
- 8 min read
Updated: Mar 4
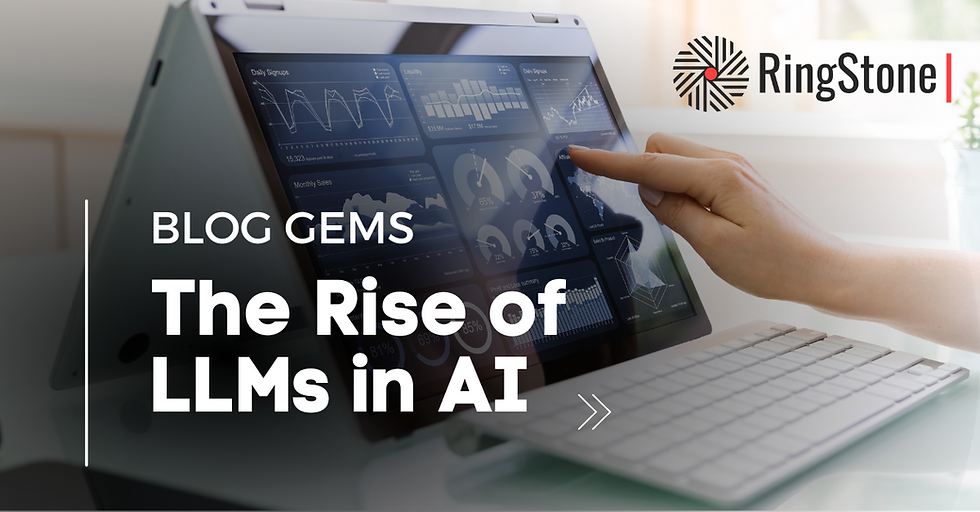
Artificial Intelligence (AI) has taken the world by storm, and the rapid advancements in this field continue to reshape industries and businesses. In a recent exploration of the AI landscape, we delve into key trends and developments driving this transformative wave.
What Is a Large Language Model?
A Large Language Model (LLM) Is A Sophisticated Artificial Intelligence System
Designed To Understand And Generate Human-Like Text At Scale
A large language model (LLM) is a sophisticated artificial intelligence system designed to understand and generate human-like text at scale. These models are typically trained on vast amounts of diverse textual data, enabling them to learn the intricacies of language, context, and patterns.
Examples Of LLMs
OpenAI's GPT-3 (Generative Pre-trained Transformer 3) has revolutionized natural language processing tasks. These large language models can comprehend context, generate coherent responses, and perform language-related tasks such as translation, summarization, and question-answering.
Microsoft's Azure Cognitive Services leverages language models for applications in business settings. Offering features like sentiment analysis, language understanding, and text analytics, these services enable businesses to gain insights from large volumes of text data.
Google Cloud's Natural Language API is another example, providing businesses with capabilities to extract information, understand sentiment, and categorize content. These commercial LLMs empower organizations by automating language-related tasks, enhancing customer interactions, and improving decision-making processes through the analysis of textual data.
The Benefits Of LLMs
LLMs Offer Businesses The Potential For Increased Productivity, Improved
Customer Engagement, And More Informed Decision-Making
The benefits that large language models offer to businesses are multifaceted:
Enhance Efficiency & Productivity: They enhance efficiency by automating tasks that involve understanding and generating human-like text, saving time and resources.
Improve Customer Engagement: Businesses can leverage LLMs for improved customer interactions, with applications in chatbots, virtual assistants, and sentiment analysis to gauge customer feedback.
Enable Data-Driven Decision Making: LLMs empower organizations to extract valuable insights from large volumes of textual data, enabling data-driven decision-making and enhancing overall competitiveness.
In essence, large language models are transformative tools that advance language-related applications, providing businesses with the potential for increased productivity, improved customer engagement, and more informed decision-making.
How Are LLMs Being Used In Business?
Large Language Models (LLMs) are increasingly used across industries to enhance business processes and applications. Here are five examples of how LLMs are being used in businesses today:
1. Chatbots for Customer Support
Many businesses employ LLMs to power conversational agents, or chatbots, for customer support. These chatbots can understand natural language queries, provide information, assist with troubleshooting, and even handle basic transactions. By using LLMs, businesses can enhance the efficiency and responsiveness of their customer support, providing instant assistance and improving user satisfaction.
2. Automated Content Generation
LLMs are employed for automated content generation in various industries, including journalism, marketing, and e-commerce. These models can produce coherent and contextually relevant text, allowing businesses to automate the creation of product descriptions, blog posts, news articles, and marketing copy. This capability streamlines content production processes and ensures a consistent tone and style.
3. Sentiment Analysis in Social Media Monitoring
Businesses use LLMs to analyze sentiment on social media and other textual data sources. By analyzing user comments, reviews, and mentions, LLMs can determine the sentiment associated with a brand, product, or service. This information is valuable for businesses to gauge customer satisfaction, identify potential issues, and adjust their strategies based on public sentiment.
4. Email Filtering and Categorization
LLMs are employed in email filtering systems to categorize and prioritize emails automatically. These models can understand the context and content of emails, allowing businesses to filter out spam, categorize emails into relevant folders, and identify urgent messages. This application improves email management efficiency for individuals and organizations.
5. Legal Document Analysis and Contract Review
LLMs are used for document analysis and contract review in the legal sector. These models can quickly analyze and extract key information from legal documents, helping legal professionals review contracts, identify risks, and ensure compliance. This application speeds up the document review process, allowing legal teams to focus on more complex and strategic aspects of their work.
The Surprising Lead Of AI
It's been over a year since the launch of ChatGPT, and its impact on the world is nothing short of remarkable. The journey of AI has surpassed previous expectations, revealing emergent behaviors that defy previous understanding.
In the not-so-distant past, AI was perceived as a promising technology confined to demos, often faltering when implemented in real-world scenarios. This perception persisted until around 2015 when major players like Google, Facebook, Uber, and Tesla cracked the code with deep learning techniques. These breakthroughs, primarily within hyperscale companies, paved the way for optimizing tasks like advertising on an unprecedented scale.
However, the real game-changer emerged around mid-last year. Major breakthroughs led to the development of larger models, unveiling emergent behaviors that defied previous understanding. Crossing certain size thresholds resulted in models exhibiting new capabilities, giving rise to the concept of foundation models. This paradigm shift drastically altered the unit economics and adoption rates of newer AI models.
Drawing Parallels With Tech Waves
The swift integration and immediate value creation resemble past revolutions like the advent of CPUs, databases, and networking. Creating new models has introduced a fourth fundamental component, potentially leading to the establishment of trillion-dollar companies in the future.
The unpredictable nature of technological evolution makes it challenging to draw precise parallels, but the consensus is that AI is becoming an integral building block for a wide array of software applications. There is a strong likelihood of a second wave, where a fundamental rethink of software from the ground up could result in a more profound impact on established players.
Shaping The Future Of Software Companies
The 2nd Wave Of AI Development Is Anticipated To Bring About More Significant
Disruptions, Challenging Incumbents & Paving The Way For New Players
The imminent question on the minds of software executives and investors is, who will shape the next evolution of AI, and what defensive moats do incumbents possess? Unlike industries with clear network effects or two-sided marketplaces, AI seems to lack deep moats. While model training costs, expertise, and proprietary data provide some advantages, the landscape remains open and competitive.
The second wave of AI development is anticipated to bring about more significant disruptions, challenging incumbents and paving the way for new players. The focus may shift from retrofitting AI into existing products to reimagining software architecture from the ground up, with models at the core.
Navigating The AI Tech Stack
Understanding the AI tech stack is crucial for companies navigating the AI landscape. The stack, reminiscent of a classic software stack, comprises silicon (dominated by Nvidia), AI clouds (offering compute capacity), and a diverse software ecosystem. Like the classic stack, the software ecosystem includes closed and open-source model providers, with companies like OpenAI leading the way.
The Continuing Quest For Model Improvement
A critical aspect of AI development revolves around the quality and quantity of data used to train models. While Large Language Models (LLMs) have made significant strides, there is an ongoing debate about the need for further improvements. Scaling models beyond current sizes faces limitations regarding data requirements and the underlying computing platform.
Efforts to optimize models for performance on existing hardware, such as leveraging techniques like mixed-precision training, sparse networks, and attention mechanisms, contribute to the ongoing quest for improvement. As the market shifts, we predict a slowdown in size increases, with the focus shifted to enhancing efficiency and performance.
Navigating Regulatory Changes
Regulating rapidly evolving tech markets poses significant challenges, and early regulatory efforts may fall short due to the dynamic nature of AI development. The need for regulation is questioned, with various concerns raised, including biased models, intellectual property, and potential threats that may be more speculative than realistic.
Creating a learning period before implementing blanket regulations is crucial, allowing time to understand AI's impact better. While regulatory frameworks are being explored globally, the consensus is that premature regulation may hinder rather than facilitate AI advancements.
Challenges In The Chip Supply Chain
The Shortage In Chip Supply, Combined With Geopolitical Uncertainties,
Underscores The Need For A Diversified And Resilient Chip Supply Chain
The global chip shortage has sent shockwaves through various industries, causing widespread supply chain disruptions. This shortage, primarily in semiconductor chips, has been fueled by increased demand, supply chain complexities, and geopolitical factors.
Beyond geopolitical risks, the industry faces GPU capacity and semiconductor manufacturing constraints. The demand for compute-intensive AI models exacerbates the situation, with potential ramifications for various sectors.
The shortage in chip supply, combined with geopolitical uncertainties, underscores the need for a diversified and resilient chip supply chain. Initiatives to enhance chip capacity globally are underway, but the complex nature of semiconductor manufacturing poses significant challenges.
The Future Of AI Pricing
It’s important to note the potential impact of supply constraints on AI pricing. While current costs remain relatively low, the demand for AI continues to surge. The efficiency gains and continuous technological advancements suggest that pricing may not see a steep rise in the near future.
Efforts to optimize model performance and explore efficiency improvements create headroom for further cost reductions. The transformative impact of AI, reducing costs by orders of magnitude, makes it unlikely for the pricing landscape to revert to previous levels.
Conclusion
The Future May See LLMs Evolving To Handle More Complex Tasks, Fostering
Advancements In Healthcare, Finance, And Scientific Research
As we navigate the uncharted waters of AI, this exploration offers valuable insights into the evolving landscape. From the surprising leaps in AI capabilities to the challenges in the chip supply chain, the journey unfolds with promises of continued innovation and transformative possibilities. As businesses and industries harness the power of AI, the key lies in adapting strategies, embracing technological advancements, and navigating the dynamic terrain of artificial intelligence.
Businesses are leveraging Large Language Models (LLMs) to revolutionize their operations. The widespread adoption of LLMs is evident in applications such as chatbots for customer support, automated content generation, sentiment analysis in social media monitoring, email filtering and categorization, and legal document analysis. These models bring efficiency, automation, and enhanced natural language understanding to diverse industries, streamlining processes and improving customer interactions.
Looking ahead, the rise in popularity of LLMs is expected to continue as advancements in natural language processing technology persist. Businesses will likely explore new use cases, expanding beyond the current applications. LLMs may play a pivotal role in personalized marketing, providing tailored content recommendations based on user preferences. Additionally, integrating LLMs in knowledge management systems could enhance information retrieval and collaboration within organizations.
The future may see LLMs evolving to handle more complex tasks, fostering advancements in healthcare, finance, and scientific research. For instance, in healthcare, LLMs could assist in analyzing medical literature, extracting insights, and supporting clinical decision-making. Moreover, with ongoing developments in multilingual models, LLMs are expected to break down language barriers and facilitate global communication and collaboration.
However, as LLMs become more prevalent, ethical considerations will remain critical, including bias mitigation and privacy concerns. Striking a balance between innovation and responsible use will be essential to ensure the ethical deployment of LLMs across industries.
The future of LLMs holds immense potential for transformative applications across diverse sectors. As businesses continue to recognize the value of these models in enhancing communication, decision-making, and automation, the trajectory points toward a future where LLMs play an integral role in shaping a more intelligent and connected digital landscape.
About The Author
With an unwavering dedication to the convergence of technology and marketing, Victoria Steed has honed her expertise over a remarkable 17-year career in the tech industry. As Director of Marketing at RingStone, Victoria seamlessly operates at the intersection of tech and marketing, driving impactful go-to-market strategies and fostering partnerships within the private equity community.
Prior to joining RingStone, Victoria played a pivotal role in the growth and success of a global consultancy in the tech sector. As the Director of Marketing, she led impactful marketing initiatives and contributed significantly to expanding the consultancy's footprint.
Beyond traditional marketing, Victoria brings a profound understanding of technology's transformative power. Her mission is to contribute to positive change through strategic marketing initiatives, one deployment and one engagement at a time. Contact Victoria at victoria.steed@ringstonetech.com.